Unfamiliar face recognition, the visual identification of a person with whom you are unfamiliar, is commonly utilized in security settings. However, our continued reliance on unfamiliar face recognition for identity verification is not supported by findings from psychological science [1]. Research has shown that whether it be for face photos or live faces, specialists or student control groups, unfamiliar face recognition is prone to error and can be exploited by fraudsters seeking to deceive identity checkers.
The selection of super-recognizers (SRs)—professionals trained in unfamiliar face recognition for security-critical roles—would appear to be the best strategy at present to improve accuracy in unfamiliar face identification. However, the selection and deployment of these individuals must be standardized, with clear criteria for SR categorization, and individual SRs must be assessed across a variety of tests (i.e., matching and memory) to ensure effective deployment.
This article will review the state of the art in unfamiliar face recognition research, before discussing two newer forms of identity fraud: hyper-realistic masks and morphs. Advancements in surveillance and biometric technologies will not obviate the need for border and law enforcement agencies to have capabilities in human-based facial recognition.
Unfamiliar Face Recognition in Security Contexts
Border control officials are required to decide whether a traveler’s passport photo matches their face, and the wrong decision in this context could result in identity fraudster entering the country. Although psychological research has already established that matching unfamiliar faces is prone to error and can be exploited by fraudsters wishing to deceive ID checkers, authorities continue to rely heavily on unfamiliar face recognition for identity verification within the national security framework [2-4].
The Glasgow Face Matching Test (GFMT) is a well-established test of unfamiliar face recognition, which mirrors the photo-to-face matching task performed by border officials [5]. Test participants are asked to decide whether pairs of high-quality face photos show the same person or two different people (see Figure 1). Accuracy on this task is poor: error rates of between 15 and 20 percent are standard, rising to 30 percent when still photographs of faces are taken from poor quality closed-circuit television (CCTV) [6]. In addition, unfamiliar face matching error rates have been shown to reach 40 percent when the faces are of a different ethnic background than the viewer (for example, in U.K./Egyptian faces arrays) [7].
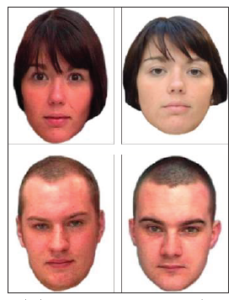
Figure 1: An example of two trials from the Glasgow Face Matching Test. The top pair shows two instances of the same person (match trial), while the bottom pair shows two different people (mismatch trial) [5]
Researchers investigated whether the inclusion of a face photo on credit cards would reduce identity fraud [10]. The study found that motivated supermarket cashiers who frequently check photo-ID cards accepted fraudulent photo credit cards (i.e., the photo did not depict the bearer) as genuine on more than 50 percent of trials.
For police officers with experience in forensic identification, error rates were found to be no better than student controls in a task that required matching unfamiliar face photos to CCTV image stills [11]—a core task in security contexts, such as border patrol and military installation base security. In addition, an international study of face recognition performance centered on Australian passport officials reported that this group incorrectly accepted a fake passport photo as genuine in 14 percent of trials—with performance on the GFMT being no different to student controls [12]. These findings suggest that training and professional experience alone do not lead to improved face recognition performance by specialists.
Super-recognizers
As previously outlined, research shows that trained and highly motivated face recognizers in law enforcement, border control, and the retail industry do not show an advantage in unfamiliar face matching performance relative to controls. However, tests of unfamiliar face recognition consistently show a wide range of individual differences in performance. Therefore, selecting SRs could hold the key to improved face matching accuracy [13].
Support for the use of SRs in security contexts has been provided by a small but growing field of literature on the SR advantage. The London Metropolitan Police utilize officers who have been tested and categorized as SRs to assist with the identification of suspects from photographs or still images captured from CCTV video. A recent review stated that individuals categorized as SRs score exceptionally well on standard tests of face recognition (such as the Cambridge Face Memory Test [CFMT]) [14,15] and tests that require both recognition memory for faces and simultaneous face matching [16]. SRs are also adept at recognizing familiar and unfamiliar faces [17,18], and eye-tracking data suggests there may be qualitative differences in the way in which SRs process faces [19].
Research on super-recognition is still in its infancy, and while the London Metropolitan Police Super-Recogniser Unit has received positive feedback from within the force, researchers outline a number of insightful caveats in relation to the research findings, as well as a series of important recommendations [14]. The authors note that when group level analyses are performed (SRs vs controls), the SRs (as a group) outperform controls. However, further analysis of individual SR scores reveal that not all are performing above average on these tests, and, in some cases, SRs can perform exceptionally well on tests of face memory (useful in identifying bad actors) but poorly on tests of face matching (such as detecting a passport photo that does not match a travelers face at border control) [16]. Therefore, SRs cannot be solely selected on the basis of face memory test performance.
The development of standardized tests for super-recognition and clear criteria for the categorization of such individuals in task related contexts (memory/matching) is recommended to further establish the utility of SR in face recognition contexts and to determine if there is a ceiling for unfamiliar face recognition. Additionally, further research is required to explore how superior SR capabilities may be utilized to bolster existing recognition algorithms [14].
Sophisticated Fraud: Hyper-Realistic Silicone Masks
It is possible for a fraudster to obtain a passport that displays a photograph of an individual who looks similar to, but is not, the fraudster. SRs have been shown effective in identifying this type of opportunistic face identity fraud. In fact, London Metropolitan Police SR’s were found to exhibit above average scores on the GFMT mismatch subtest [20], which is analogous to this type of fraud. However, it is not clear whether SRs would display an advantage in the detection of deliberate disguise, in which a fraudster implements physical items such as glasses, wigs, and beards to physically alter their appearance in order to look more like the person in the stolen passport photo. It is important to note that deliberate disguise facilitates a direct route to identity fraud.
In 1994, researchers assessed the effects of using eyeglasses and beards as disguises [21]. Individuals who participated in this research studied a series of faces which included these disguises. Results indicated that when beards were removed recognition of the face was reduced by 30 percent, and when eyeglasses were removed, recognition was reduced by 40 percent. Similarly, a 2014 study determined that participants accepted two different, but disguised faces, as the same person 19 percent of the time when subjects are of the same ethic background, and 24 percent of the time for subjects of a different ethnic background [22].While these single item physical disguises provide a route to identity fraud, fraudsters are seeking sophisticated disguises, such as hyper-realistic, over-head silicone face masks.
These silicone masks cover the neck and chest so that clothing can obscure the edges of the silicone. They are produced by a small number of companies and are used primarily in the entertainment industry. However, in a recent string of U.S. bank robberies, a white offender used one of these masks to disguise himself as black [23]. In a photo line-up, six out of the seven bank tellers who witnessed the robberies wrongly identified the culprit as black because they accepted the mask as a genuine face. The situation was only resolved when the girlfriend of the actual offender notified the police, at which point, the detained African American suspect was released from jail [23]. In another notable example, a young Asian man had obtained the passport of an elderly white Caucasian male [24]. Using a hyper-realistic mask, he was able to pass several identity checks at a Hong Kong airport. The use of the mask was only discovered when the perpetrator decided to remove it mid-flight [24]. Such reports represent a concerning new route to identity fraud, in which an individual can completely change their facial appearance to match stolen identification documents.
Despite the use of these masks by fraudsters, only one study assesses the ability to detect the presence of/be fooled by these masks [25]. In the study, a white Caucasian man wore a hyper-realistic white Caucasian mask, as seen in Figure 2, and sat on a bench in the middle of a university campus reading a book [25]. Passers-by were stopped and asked to rate the individual on task-irrelevant dimensions, such as attractiveness, from a distance of 5 meters (m) (near) or 10 m (far). Upon completing the rating, participants were then asked a spontaneous, prompted, or explicit mask-detection question. The study found that none of the participants in the far condition, and only 6 percent in the near condition reported the presence of a mask at spontaneous or prompted questioning. For the explicit report question—“Was that person wearing a hyper-realistic mask?”—57 percent of participants failed to detect that the man was wearing a mask. Detection rates were significantly higher for those viewing from 2 m than 10 m. This study shows that in a naturalistic context, with relatively close viewing distances, mask detection rates are low.
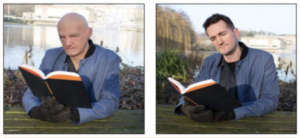
Figure 2: An illustration of the live mask detection experiment set in the middle of a university campus. The images show the participant
wearing the hyper-realistic mask (Left) and the participant without the mask (Right) [25] (Released).
Internet and smartphone users now have access to a variety of face image manipulation apps that support the digital morphing of face photos. These apps can take two different photos of faces and merge (morph) them to become a new photo that retains facial information that is specific to both identities (see Figure 3 for examples). Identity fraudsters can utilize this digital tool in two ways. First, if a fraudster has a willing participant, a passport morph could fool a passport renewal official (as it looks somewhat like the participant’s file photo) into issuing a fraudulently obtained genuine (FOG) passport, which both individuals can use [26-28]. Secondly, even without access to a participant, fraudsters can use stolen identity information to complete the same process to obtain a FOG passport for their own use.
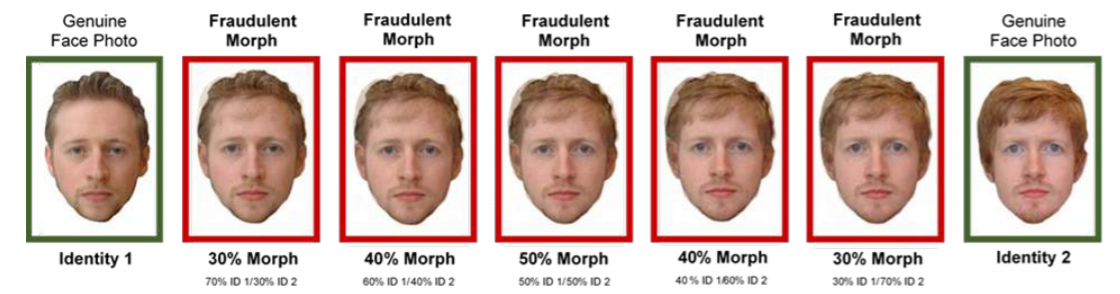
Figure 3: Examples of the morphed passport photos [29]
Conclusion
While the selection and recruitment of SRs is a welcome advance in improving the accuracy of unfamiliar face identification, the standardization of testing and selection criteria as well as subsequent effective deployment are key further considerations. Additionally, further research is required to ensure the development of effective counter-measures against this type of sophisticated fraud, such as enhancing machine learning techniques by integrating lessons learned from SRs. The Department of Defense may benefit from this research as border patrol and military installation base security sometimes still visually inspect persons and their corresponding identification to prevent unauthorized access. The aforementioned face recognition techniques could also be used for identification of individuals during surveillance missions and in body identification.
References
1. Burton, A. M. (2013). Why has research in face recognition progressed so slowly? The importance of variability. Quarterly Journal of Experimental Psychology, 66, 8,1467-1485.
2. Jenkins, R. & Burton, A.M. (2011). Stable face representations. Philosophical Transactions of the Royal Society of London, B, 366, 1671-1683.
3. Robertson, D. J., & A. Mike Burton. (2016). Unfamiliar face recognition: Security, surveillance and smartphones. Journal of the U.S. Homeland Defense and Security Information Analysis Center, 3(1), 14-21.
4. Robertson, D. J., Middleton, R., & Burton, A.M. (2015). From policing to passport control. The limitations of photo ID. Keesing Journal of Documents and Identity, 46, 3-8.
5. Burton, A. M., White, D., & McNeill, A. (2010). The Glasgow Face Matching Test. Behavior Research Methods, 42(1), 286-291.
6. Bruce, Henderson, Newman, Burton. (2001). Matching identities of familiar and unfamiliar faces caught on CCTV images. Journal of Experimental Psychology: Applied, 7(3) doi:10.1037%2F1076-898X.7.3.207
7. Megreya, A. M., White, D., and Burton, A. M. (2011). The other-race effect does not rely on memory: Evidence from a matching task. The Quarterly Journal of Experimental Psychology, 64(8), 1473-1483. doi: 10.1080/17470218.2011.575228
8. Megreya, A.M. & Burton, A.M. (2008). Matching faces to photographs: Poor performance in eyewitness memory (without the memory). Journal of Experimental Psychology: Applied, 14, 364-372.
9. Davis, J. P., and Valentine, T. (2009). CCTV on trial: Matching video images with the defendant in the dock. Applied Cognitive Psychology, 23(4), 482-505.
10. Kemp, R. I., Towell, N., and Pike, G. (1997). When seeing should not be believing: Photographs, credit cards and fraud. Applied Cognitive Psychology, 11(3), 211–222.
11. Burton, A. M, Wilson, S., Cowan, M., and Bruce, V. (1999). Face recognition in poor quality video: Evidence from security surveillance. Psychological Science, 10, 243-248.
12. White, D., Kemp, R.I., Jenkins, R., Matheson, M., and Burton, A.M. (2014). Passport Officers’ Errors in Face Matching. PLoS One, 9(8).
13. Robertson, D.J. (2018). Could super recognisers be the latest weapon in the war on terror? Retrieved from https://theconversation. com/could-super-recognisers-be-thelatest-weapon-in-the-war-on-terror-56772.
14. Noyes, E., & O’Toole, A. J. (2017). Face recognition assessments used in the study of super-recognisers. Retrieved from https://arxiv.org/abs/1705.04739v1.
15. Russell, R., Duchaine, B., & Nakayama, K. (2009). Super-recognizers: People with extraordinary face recognition ability. Psychonomic Bulletin & Review, 16, 252–257.
16. Bobak, A., Hancock, P. J. B., & Bate, S. (2016). Super-recognisers in action: Evidence from face-matching and face memory tasks. Applied Cognitive Psychology, 30, 81–91.
17. Davis, J. P., Lander, K., Evans, R., & Jansari, A. (2016). Investigating predictors of superior face recognition ability in police super-recognisers. Applied Cognitive Psychology, 30, 827–840.
18. Robertson, D. J., Noyes, E., Dowsett, A. J., Jenkins, R., & Burton, A. M. (2016). Face recognition by Metropolitan Police super-recognisers. PLOS One, 11(2), 1-8. doi: 10.1371/journal.pone.0150036
19. Bobak, A. K., Parris, B. a, Gregory, N. J., Bennetts, R. J., & Bate, S. (2016). Eye movement strategies in developmental prosopagnosia and “super” face recognition. Quarterly Journal of Experimental Psychology, 70, 201–217.
20. Robertson, D. J., & A. Mike Burton. (2016). Unfamiliar face recognition: Security, surveillance and smartphones. Journal of the U.S. Homeland Defense and Security Information Analysis Center, 3(1), 14-21.
21. Terry, R. L. (1994). Effects of facial transformations on accuracy of recognition. Journal of Social Psychology, 134(4), 483492. doi: 10.1080/00224545.1994.9712199
22. Dhamecha TI, Singh R, Vatsa M, Kumar A (2014) Recognizing disguised faces: Human and machine evaluation. PLOS ONE, 9(7): e99212. doi: 10.1371/journal.pone.0099212
23. Bernstein, S. (2010). Masks so realistic they’re arresting the wrong guy. Retrieved from http://articles.latimes.com/2010/dec/08/business/la-fi-mask-20101209.
24. Zamost, S. (2010). Exclusive: Man in disguise boards international flight. Retrieved from http://edition.cnn.com/2010/WORLD/americas/11/04/canada.disguised.passenger/index.html.
25. Sanders, J. G., Ueda, Y., Minemoto, K., Noyes, E., Yoshikawa, S., & Jenkins, R. (2017). Hyper-realistic face masks: A new challenge in person identification. Cognitive Research: Principles and Implications, 2(1). doi: 10.1186/s41235-017-0079-y
26. Middleton, R. (2014). For terrorists, documents are as important as weapons. CSEye: Journal of the UK Forensic Science Society, 1(2), 6-10.
27. U.K. HM Passport Office Report: Basic Passport Check. 2015. Retrieved from https://www.gov.uk/government/publications/basic-passport-checks.
28. U.K. National Fraud Authority Report: Fighting Fraud Together. (2011). Retrieved from https://www.gov.uk/government/uploads/system/uploads/attachment_data/file/118501/fighting-fraud-together.pdf.
29. Robertson, D., Kramer, R., & Burton, M.(2017). Fraudulent ID using face morphs: Experiments on human and automatic recognition. doi: 10.1371/journal.pone.0173319